Quantum machine learning quantum autoencoder can eliminate the noise of quantum measurement
Quantum machine learning quantum autoencoder can eliminate the noise of quantum measurement
Quantum machine learning quantum autoencoder can eliminate the noise of quantum measurement
Many research groups around the world are currently trying to develop instruments that can collect high-precision measurements, such as atomic clocks or gravity meters. Some of these researchers try to achieve this goal using entangled quantum states, which are more sensitive than classical or non-entangled states. However, due to this high sensitivity, the quantum entangled state is also easier to pick up noise from irrelevant signals when collecting measured values, which hinders the development of accurate and reliable quantum-enhanced metrology equipment.
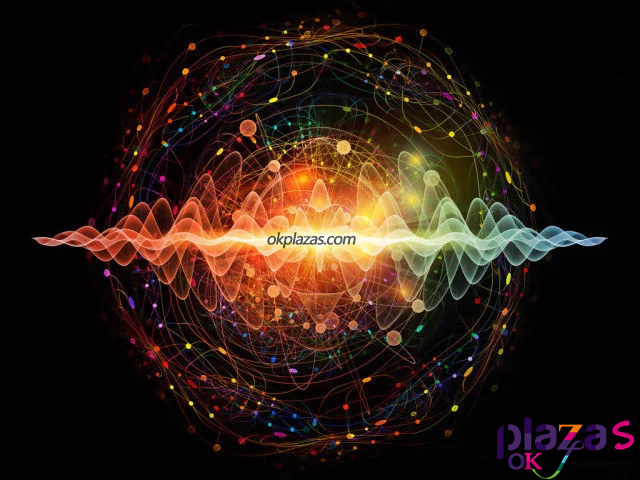
To overcome this problem, researchers at Leibniz University in Hannover, Germany have recently developed a quantum machine learning algorithm that can be used to denoise quantum data. This algorithm can help use quantum clocks or other measurement tools based on entangled quantum states. Produce more reliable data. Their research results were published in the recent Physical Review Letters
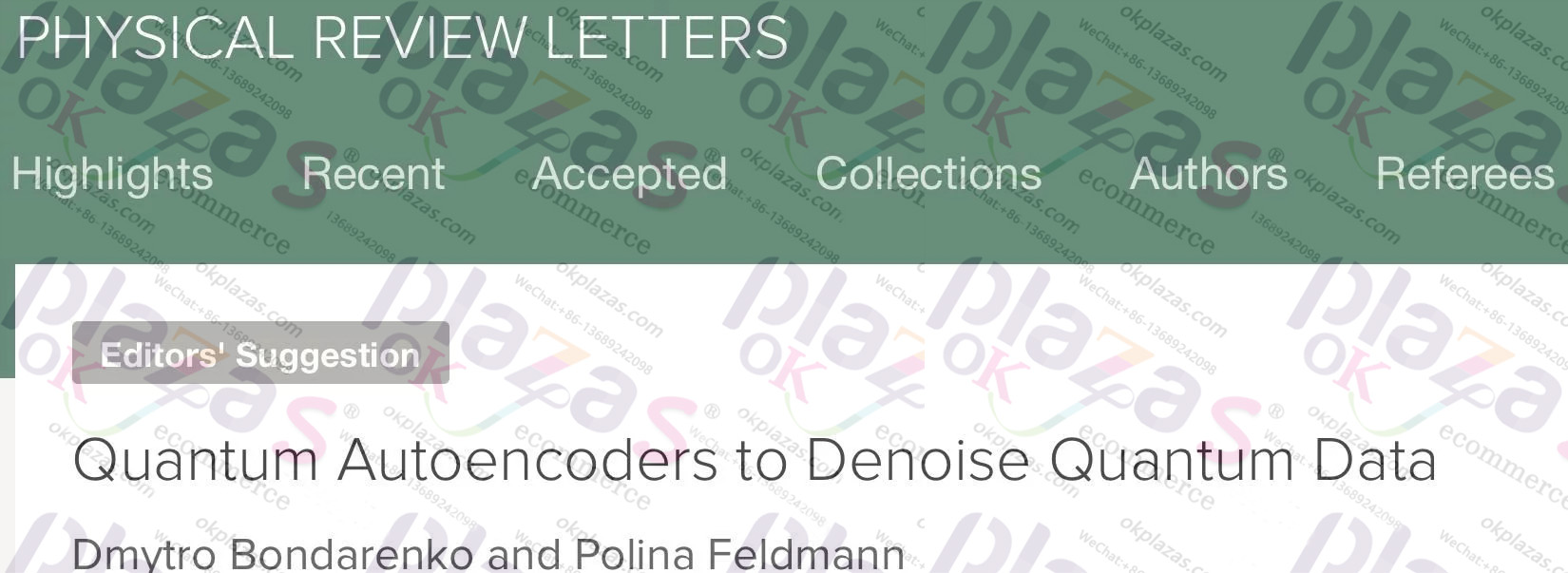
Machine learning is a universal method of data analysis. Quantum machine learning combines the versatility of machine learning with the functions of quantum algorithms. It is a very promising method. Like traditional machine learning, quantum machine learning algorithms rely on a series of variational parameters. These parameters need to be optimized before the algorithm can be used to analyze the data. In order to learn the correct parameters, the algorithm must first be trained. The algorithm is related to the task to be completed by the design, such as pattern recognition and image classification.
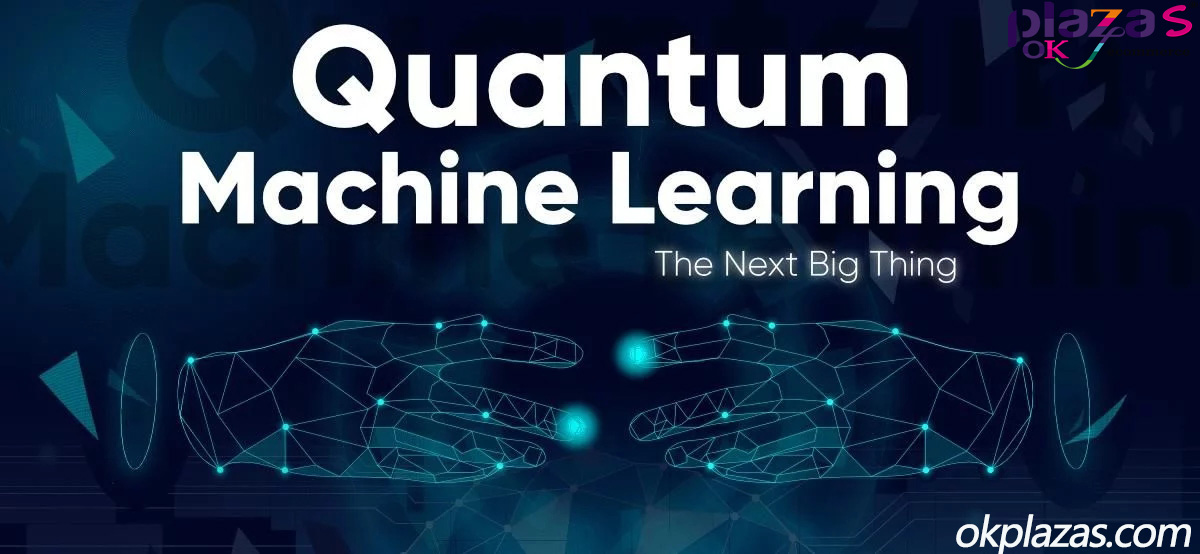
For quantum machine learning, the input and output of an algorithm are quantum states. For example, a quantum state can be realized by using a superconductor. The algorithm that maps the input state to the output state is implemented on a quantum computer. The variational parameters that must be optimized are the classical parameters of the transformation performed on the quantum computer.
Researchers want to test whether the previously developed quantum machine learning algorithms can be used to clean up data collected using quantum-enhanced measurement tools, which ultimately led to the development of the quantum autoencoder described in their paper. The researchers explained: "Suppose there is a quantum experiment, which gives a lot of noisy quantum states." "In addition, suppose there is a quantum computer that can handle these states. Our autoencoder is an algorithm that can tell quantum computers. How to convert the noisy quantum state in the experiment into a denoising state."
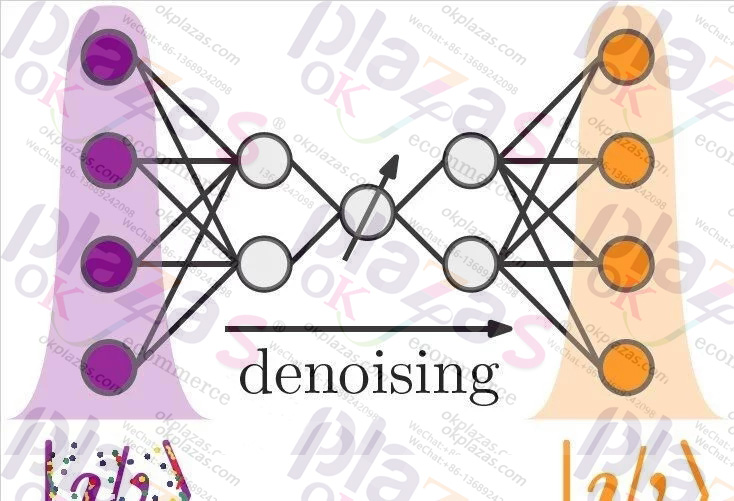
The researchers first optimized their algorithm and trained it to effectively denoise quantum data. Since the denoising reference state is difficult to obtain or cannot be obtained through experiments, the researchers used a technique that is usually used when optimizing the classic autoencoder, which is an unsupervised machine learning algorithm.
The researchers said: "The trick is that the algorithm must be written in a way that reduces the information from the input to the output state." "Now, the quality factor is defined as the similarity between the state processed by the autoencoder and another noisy state in the experiment. In order to make these states as similar as possible, the autoencoder must maintain the same information in the two states, that is, their common silent source, while the noise is different in each state produced by the experiment."
The researchers performed many simulations in which noisy entangled quantum states were produced. First, they used these "experimental" outputs to optimize the changing parameters of the autoencoder. Once the training phase is completed, the performance of its autoencoder in denoising quantum measurements can be evaluated.
The researchers said: "The advantage of our method is that it is universal." "There is no need to know the experimental output in advance, nor to characterize the noise source. Even if the experimental output is not unique, it depends on some experimental control parameters, and the denoising is still measured The application works."
The purpose of the numerical experiment is to denoise some highly entangled quantum states with spin flip errors and random cell noise. Their algorithm has achieved remarkable results and can also be implemented on current quantum devices.
These algorithms require a quantum computer that can process the output quantum data of a specific experiment. For example, if researchers are trying to use autoencoders to denoise data based on captured ions, but quantum computers use superconducting qubits, they also need to use a technology that can map states from one physical platform to another. .
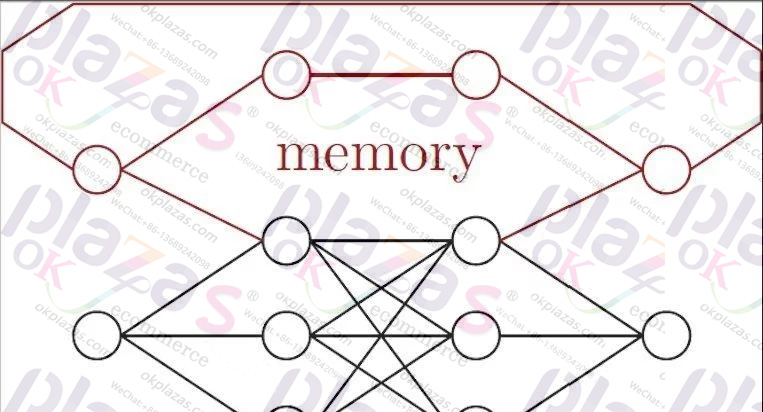
Although quantum machine learning techniques and quantum computers have been found to perform well in various tasks, researchers are still trying to determine the practical applications for which they may be most useful. Their recent research provides a clear example of how quantum machine learning methods can finally be effectively applied to the real world.
The developed quantum autoencoder can be used to improve the reliability of measurement results collected using quantum enhancement tools, especially tools that use multi-body entangled states. In addition, it can also be used as an interface between different quantum architectures.
Different quantum devices have different advantages. For example, it may be easier to use cold atoms to measure gravity, photons are very useful for communication, and superconducting qubits are more useful for quantum information processing. To convert the information exchanged between these different platforms, interfaces are needed, and these interfaces themselves introduce noise. Auto-encoders can help reduce noise in the exchanged data.
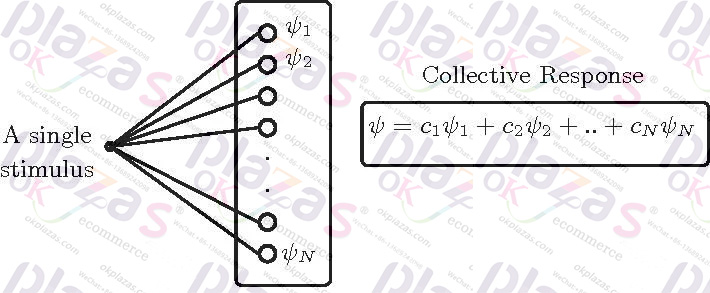
Researchers are trying to develop another type of quantum algorithm: recursive quantum neural network. The circular architecture of this new algorithm should enable it to store information processed in the past and have "memory", which will allow researchers to correct for drift. This can make quantum experiments easier, because drift will be filtered out through post-processing. Another application of recurrent neural networks is to reduce noise when the noise changes slowly. For example, if entangled photons are sent through the air, the noise may be different in different weather conditions on a snowy cloudy day and a hot day. However, the weather cannot change instantly, so an algorithm with memory is better than an algorithm without memory.